How can we enable teams to move quickly to deliver solutions andaddress good data governance in the process? Start by taking in the whole picture.
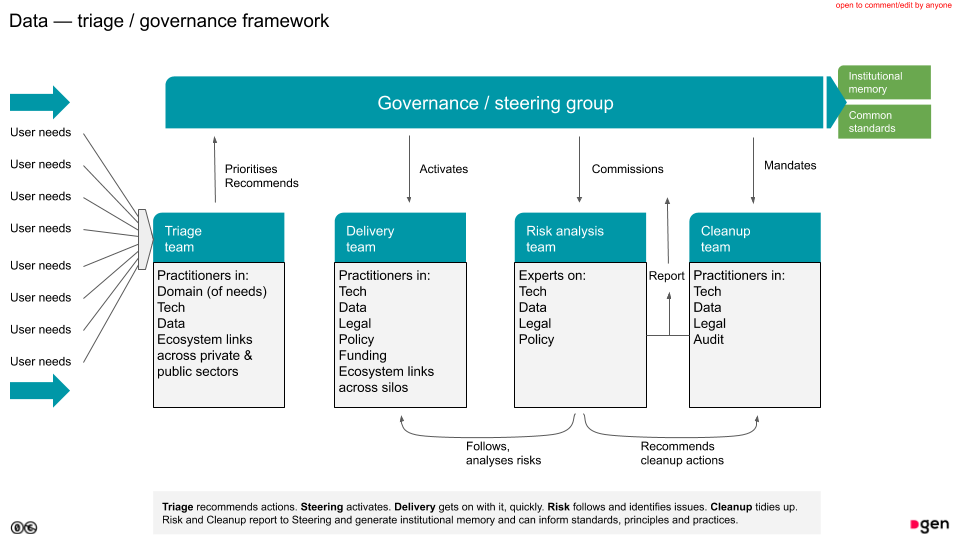
I’ve been receiving a lot of calls this year from people asking how they can use data to ‘help’ in various situations (from climate to COVID).
To help frame a potential response that isn’t just “we can build an app for that” (that’s very rarely the answer) or “we should build a portal” (that’s almost never the first-order answer), I’ve been trying to encourage people to first look at user needs, examine prior work and look at the whole system.
In times of an emergency, there’s a rush for people to want to ‘do something’ — let’s harness that energy on building useful, repeatable, stackable things rather than squander it on ‘solutionising’?
What is also apparent is that
even without an ‘emergency’, there is often a rush to technology-led solutions without considering the overall decision-making environment that will impact users.
The image above is a provocation: a potential process to ask how we can try and focus on what matters; that can unlock rapid-response from a frontline delivery team who can focus on solutions; that can pick up the pieces to address risks and harms.
Let’s also avoid just breaking things in the process (‘technical debt’ can lead to very bad outcomes) and give the frontline team (and the rest of us) confidence that there is a process following them that will tidy up, pick up the pieces and make sure we don’t leave a mess/risks.
Questions to consider:
- What problems are we really trying to solve — based on actual user needs?
- How will data help these problems?
(what data? when? what frequency is timely? what analysis? for whom?) - Who should act to convene and lead: a sector, public body, both?
- When are solutions needed and what might be our MVP (minimum viable proposition) intervention? (nb: this may not need any data at the outset)
- What single-issue could act as an exemplar to focus everyone’s mind?
- What institutional memory, standards, principles and practices can be created as a legacy output and how will we make it discoverable?
Roles & responsibilities
Steering = combined expertise to ensure decisions are timely, effective, comprehensive and address risk
Domain = sector or topic specialism that is based on the user-needs
Tech = understanding everything from coding to devops
Data = understanding data analytics, data science and algorithmic modelling
Ecosystem links = connecting across silos (public, private and third sectors)
Legal = expertise in the Domain and in data rights and ethics
Policy = expertise in the Domain and across relevant applications
Audit = expertise across all of the above to ensure ‘clean up’ is comprehensive
This article is based on an original post from April 2020.